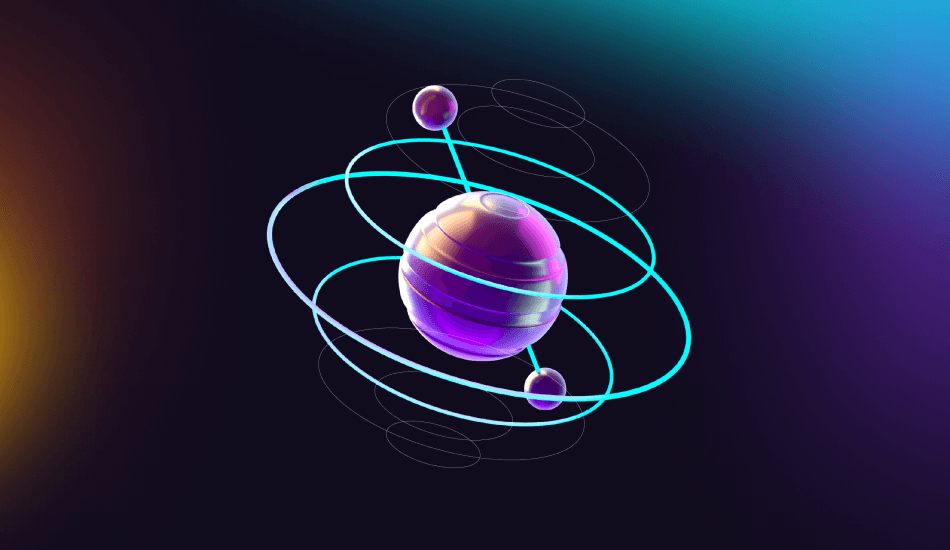
Quantum computing in 2024: The State of Play
What is quantum computing, exactly? Why should we care? And where does the cloud fit in? Have no fear, our in-house expert is here…
In the ever-evolving landscape of Quantum Machine Learning (QML), the Perceval Quest emerged as an exciting initiative to explore the synergy between classical ML and photonic quantum computing. Co-organized by Quandela and Scaleway, this competition challenged participants to revisit the classification task of the MNIST digit dataset by leveraging Perceval, Quandela’s quantum computing framework, alongside Pytorch to empower classical machine learning mechanisms.
Unlike traditional competitions seeking merely to maximize accuracy, Perceval Quest invited participants to explore novel hybrid quantum-classical architectures, pushing the boundaries of what’s possible when quantum computing principles enhance traditional machine learning methods. The challenge attracted 64 international teams, showcasing the potential of these new architectures and original methods to evaluate their utility.
"The MNIST digit classification task has been the witness and the benchmark of the evolution of classical neural networks for 30 years. While Quantum Machine Learning is emerging as a new active research field, we thought that this challenge was a good way to pay homage to this spirit and also to anchor QML development in the same tradition of benchmarking that made possible AI as we know it today" – Jean Senellart, CPO at Quandela
QML sits at the frontier of two transformative fields: quantum computing and artificial intelligence. It explores how quantum phenomena, like superposition, entanglement, and high-dimensional Hilbert spaces, can be harnessed to enrich and potentially accelerate machine learning tasks. In particular, photonic quantum computing offers unique expressiveness and parallelism, enabling novel data encodings and circuit designs that would be hard to replicate classically.
Research into QML is not just about seeking quantum advantage, it’s about rethinking how we design learning algorithms and new data expressivity, blending quantum resources with classical methods to create new hybrid paradigms.
QML has gained special relevance in the current Noisy Intermediate-Scale Quantum (NISQ) era, as we introduced in this article. With large-scale, fault-tolerant quantum computers still on the horizon 2029-2032, researchers are turning to hybrid algorithms that combine classical processing with the capabilities of today’s noisy quantum devices. These NISQ-compatible algorithms are especially well-suited for machine learning, where probabilistic reasoning and model generalization can tolerate some noise and imprecision (See Figure).
In this context, QML provides a pragmatic pathway to start leveraging quantum advantages in real-world applications, even with the hardware limitations of today's system.
Competitions like the Perceval Quest serve as valuable test places, helping the quantum community to explore these hybrid models, benchmark performance, and inspire the next generation of quantum-enhanced AI systems. After a trial of 3 months where 135 fellows among 64 teams from 12 countries, we are thrilled to unveil the top three teams that showcased the most innovative and effective approaches:
🥇 1st Place: Quantum Tree
🥈 2nd Place: Quantum Nomad
🥉 3rd Place: Solal
Mention to organization teams who also participated to the challenge (without ranking):
Beyond the competition, the insights and creative strategies developed by participants deserve to be shared with the quantum community. To that end, Quandela is preparing a dedicated scientific paper that will highlight the most promising approaches explored during the challenge.
This upcoming publication will not only showcase the diversity of techniques applied to hybrid quantum / classical learning but also provide valuable perspectives on photonic quantum computing’s role in Machine Learning tasks.
The lessons learned from this collective effort are just the beginning of a larger conversation around Quantum Machine Learning!
From January to March 2025, Scaleway QaaS played an important role in enabling participants to experiment with photonic quantum computing based on an GPU-optimized version of Perceval. Over the course of the competition, more than 150 simulated QPU sessions were launched, with the NVIDIA L4 GPUs emerging as the preferred choice for accelerated emulation. In total, the participants leveraged over 20,000 minutes of quantum computing time, pushing the boundaries of hybrid AI-Quantum models.
Since its launch during ai-Pulse 2023, QaaS has facilitated over 650 QPU sessions and executed more than 30,000 jobs, making it a key resource for researchers and developers prototyping exploring applications of quantum computing.
"We are proud that QaaS powered so many quantum explorations during the Perceval Quest. We are looking forward to enhancing our infrastructure for future challenges and larger use cases as the field is fast growing." – Valentin Macheret, R&D Engineer at Scaleway
In the upcoming months, Scaleway QaaS will continue to evolve and host more QPU platforms. One of them is the latest photonic computer Belenos from Quandela, with 12 photons into 24 modes, pushing forward quantum computing accessibility.
Through this competition, we have gathered valuable insights to refine and improve quantum frameworks and tools. First, Quantum Machine Learning has proven to be an excellent gateway into quantum computing, providing an intuitive yet powerful introduction to this cutting-edge field. However, we also recognize the need to enhance our platform by introducing better batch-processing capabilities, ensuring that large-scale experiments can run more efficiently.
Furthermore, the bridge between classical and quantum paradigms must be strengthened with improved tooling such as the newly started MerLin by Quandela. This will allow AI researchers to seamlessly integrate quantum machine learning models into their workflows and will be instrumental in building strong benchmarks to guide the evolution of Quantum Machine Learning.
Finally, optimized GPU-based emulation remains a critical asset, significantly accelerating prototyping before deploying workloads on physical QPUs.
These insights will shape the future evolution of Scaleway QaaS, as we strive, alongside Quandela, to provide a robust and scalable quantum computing environment for all.
What is quantum computing, exactly? Why should we care? And where does the cloud fit in? Have no fear, our in-house expert is here…