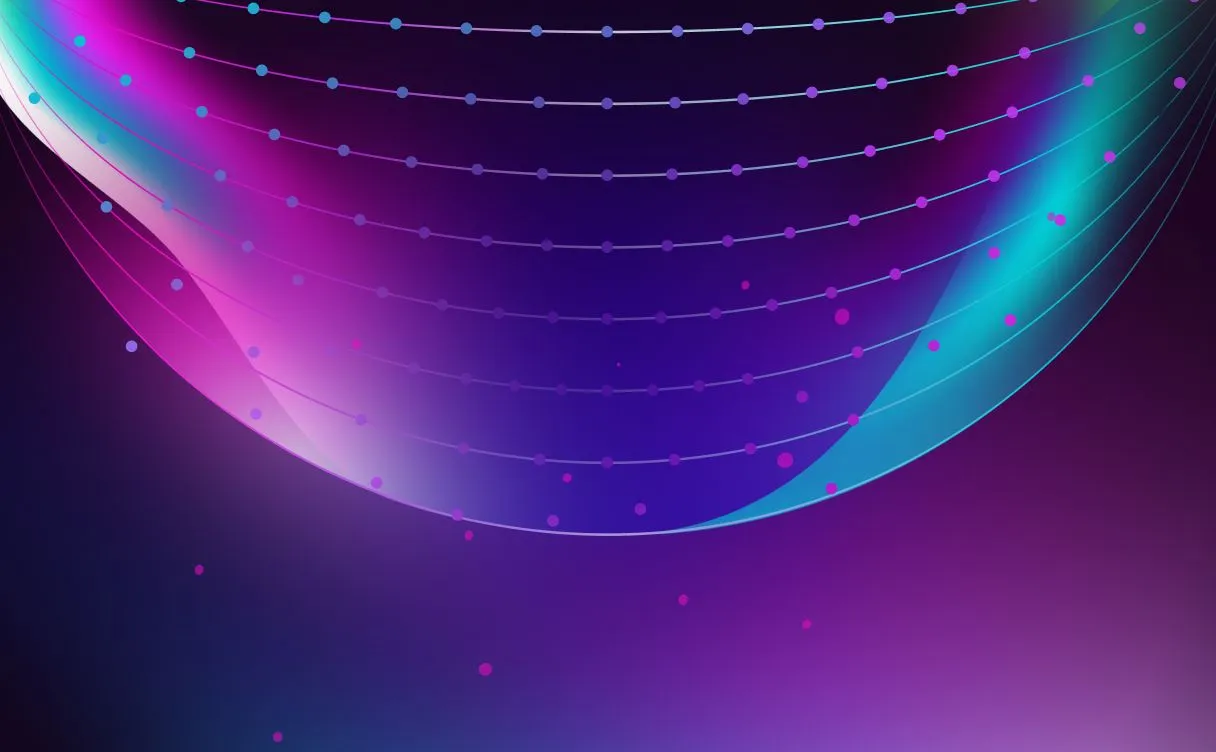
AI and the quality conundrum, with Giskard.AI’s Alexandre Combessie
In a world where AI has become more and more a common presence in our lives, the quest for quality AI solutions has taken center stage. We sat down with Alexandre Combessie, co-founder and CEO of Giskard.AI - which notably exhibited at ai-PULSE last month - to delve into the challenges around ethics and quality faced by AI solutions and their users.
With a background steeped in AI expertise, Alexandre brings a wealth of experience to the table. Before creating Giskard.AI, he spent five years at Dataiku, focusing on building models for various industries, particularly in NLP (natural language processing) and time series. His experience in crafting models for large-scale enterprises, including in healthcare and financial services, laid the foundations for Giskard.AI’s later innovative work.
Today, Giskard is a French firm that specializes in AI quality, which Combessie co-founded in 2021. It ensures AI professionals maximize Machine Learning (ML) algorithm quality, minimizing errors, biases and vulnerabilities. Giskard is as such notably establishing itself as the leading software platform for aiding compliance with upcoming AI regulations and standards.
Quality: The multifaceted essence of AI
Now that conversing with AI has become commonplace, the distinction between a run-of-the-mill AI and a quality-driven one has never been more important. Combessie emphasizes that quality in results spans multiple dimensions, with two key factors standing out:
Generative AI's hallucinations
At the heart of generative AI lies the ability to create and construct, often leading to intriguing "hallucinations," whereby the AI conjures up information that is false, leading to a range of significant issues. Such fabrications could contribute to the spread of fake news, error of diagnosis, and heighten the risk of poor human decision-making. Moreover, the possibility of errors in critical areas like medical diagnoses due to AI-generated inaccuracies is a particularly concerning aspect. Alexandre encourages us to explore hallucinations even further than has been established to date, to understand both their potential and limitations.The ethical challenge
The ethical dimension of AI looms large: the algorithms that fuel AI models are derived from existing datasets, potentially perpetuating biases and prejudices. The crucial question arises: could an algorithm be toxic, or offensive? This challenge of ethics and bias calls for profound scrutiny. Even before generative AI’s recent exponential growth, quality concerns were evident, spanning ethical biases in scoring algorithms, lack of transparency regarding AI models’ decisions, and performance issues in production.
Ethical biases in consumer applications like facial recognition have already been unearthed, and in the industrial sphere, predictive maintenance or fraud detection could prove particularly sensitive to AI’s potential mistakes. To investigate such cases, drawing on two years of dedicated quality work pre-ChatGPT, Giskard.AI was able to formulate and test diverse solutions that extend beyond chatbots to various business applications of AI, such as tabular data.
Stepping into a maturing market: ethics, risk, and performance
A key hurdle in AI's journey toward quality and ethics is the market's maturity. Concepts like risk, ethics, and performance are relatively new to the AI world, demanding both internal team education and external regulation. The importance of evangelization is at the center of those changes, and not just within a company, but also in terms of regulatory compliance. The objective is clear: minimize errors, offense, and legal concerns for high-risk AI models, which are starting to impact all we see and read.
Combessie's engineering background parallels his dedication to ensuring quality in AI. Drawing a captivating analogy to civil engineering, he emphasizes the high standards that underlie his work. He envisions building a bridge between data scientists and those who grasp the significance of AI's ethical and quality dimensions.
Beyond the accuracy of a result lies the model metrics. Which metrics truly matter for a model to be seen as a great one? Combessie rejects the notion of relying on one single KPI. Such an approach may provide limited accuracy and overlook important aspects of model performance.
The concept of "robustness metrics" emerges as a vital topic, especially for models deployed in production environments. Combessie shares a compelling example from the real estate sector, where AI-driven decisions led to catastrophic financial losses. Zillow deployed an AI algorithm to predict the prices of the homes they would sell. After having put the model in autopilot mode, they lost over $500 million in six months. They stopped trading, and fired their entire data science team. Ensuring AI models do not lead to such disastrous outcomes is a critical aspect of maintaining robustness.
Shaping Ethical AI
The responsibility for ethical AI lies with the companies that develop and deploy it. If a company's AI lacks ethics or perpetuates discrimination, that company is legally accountable. In high-risk AI scenarios, failure to adhere to ethical standards could result in fines of up to 6% of a company's revenue, according to the upcoming EU AI Act.
As stated on Giskard.AI’s blog, under the AI Act (which was passed early December), generative foundation models like ChatGPT will be subject to strict controls. These include transparency, or public disclosure of which content was created by AI; declaring what copyrighted data was used in training; and barriers to stop such models generating illegal content.
With these constraints in mind, Giskard.AI empowers companies to adhere to legislation by simplifying and measuring their compliance efficiently. As such, Giskard.AI has taken the lead in advancing ethical AI. It provides the tools to assess discriminatory biases in models, particularly concerning attributes like age, gender, and ethnicity. Collaborating with organizations like AFNOR, Giskard.AI contributes to setting standards that safeguard against biases.
Conclusion
For Combessie and Giskard, moving forwards, the key will be finding the ideal balance between innovation and regulation. "Having testing systems for ML models which are easy to integrate by data scientists are key to making the compliance process to the regulation as easy as possible," says Combessie, "so that regulation is possible without slowing down innovation, but also respecting the rights of citizens."
Furthermore, as Giskard does AI "in an open-source way", he adds, "our methods are transparent and auditable".
About Giskard
Giskard is a French software publisher specializing in Artificial Intelligence (AI) quality. Founded in 2021 by three AI experts, including two former engineers from Dataiku and a former data scientist from Thales, Giskard's mission is to help AI professionals ensure the quality of their algorithms. It assists in avoiding the risks of errors, biases, and vulnerabilities in AI algorithms.
Giskard is backed by renowned investors in the AI field, including Elaia and the CTO of Hugging Face. In August 2023, Giskard received a strategic investment from the European Commission to establish itself as the leading software platform for facilitating compliance with the European AI regulation.
Learn more: Giskard.AI