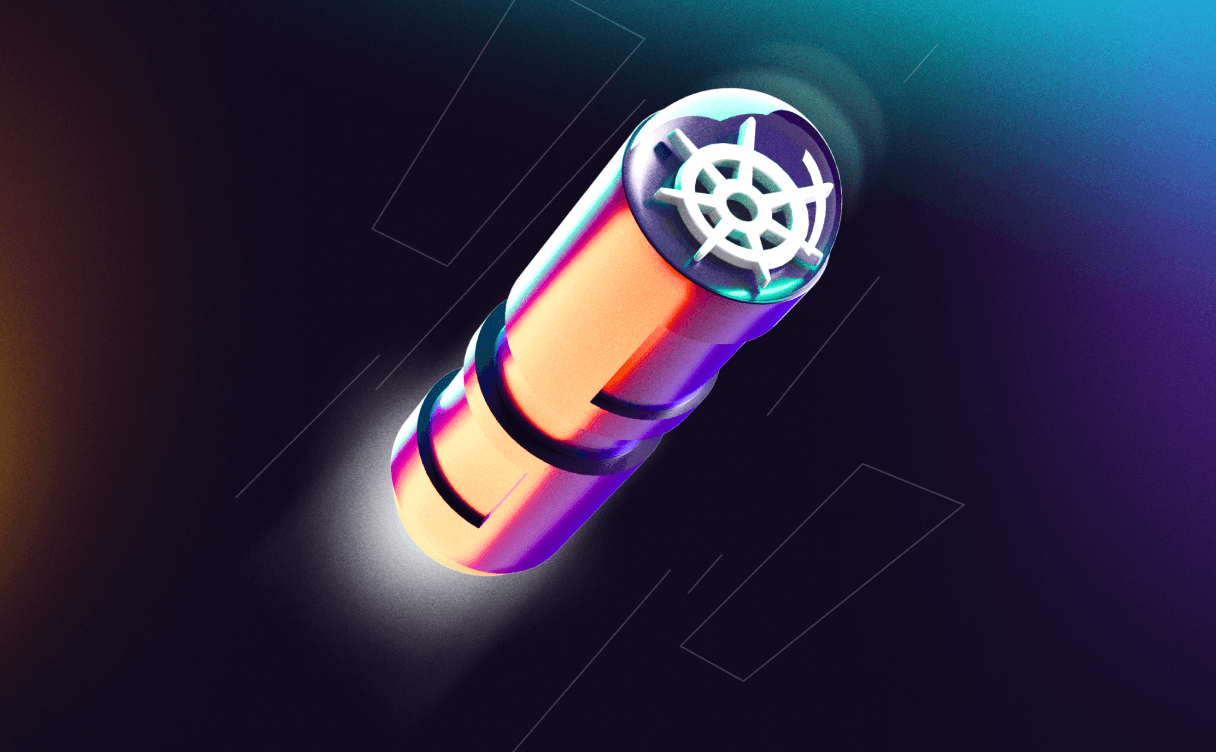
Kubernetes Kapsule to optimize costs
Deploy and Scale your infrastructure with Kubernetes.
Our comprehensive lineup of NVIDIA GPUs, including NVIDIA H100 Tensor Core, NVIDIA L40S, NVIDIA L4 Tensor Core, NVIDIA P100, and NVIDIA GH200 covers a wide range of computing needs. Harness the speed and efficiency of GPUs for parallelized workloads thanks to instances or supercomputers.
€2.73/hour (~€1,992/month)
Accelerate your model training and inference with the most high-end AI chip of the market!
€1.24/hour (~€905/month)
Dedicated Tesla P100s for all your Machine Learning & Artificial Intelligence needs.
€0.75/hour (~€548/month)
Optimize the costs of your AI infrastructure with a versatile entry-level GPU.
€1.4/hour (~€1,022/month)
Accelerate the next generation of AI-enabled applications with the universal L40S GPU Instance, faster than L4 and cheaper than H100 PCIe.
Available soon
The H200’s larger and faster memory accelerates generative AI and LLMs with better energy efficiency and lower total cost of ownership.
Available soon
Featuring 6 different technologies, the Blackwell GPU architecture enables breakthroughs in data processing, quantum computing, and generative AI.
Available now
Designed to handle the most demanding AI workloads, the AMD Instinct™ MI300 Series accelerators deliver high-speed compute performance and large memory density with high bandwidth.
GPU | GPU memory | FP16 peak performance | Recommended for | Price |
---|---|---|---|---|
2x NVIDIA H100 Tensor Core GPU | 80GB | 3,026 TFLOPS | 70B LLM Fine-Tuning / Inference | €5.04/hour |
1x NVIDIA H100 Tensor Core GPU | 80GB | 1,513 TFLOPS | 7B LLM Fine-Tuning / Inference | €2.52/hour |
8x NVIDIA L40S GPU | 48GB | 2,896 TFLOPS | Fine-Tuning / Inference of GenAI (image video) model up to 70B | €11.2/hour |
4x NVIDIA L40S GPU | 48GB | 1,448 TFLOPS | Inference of Mixtral 8x22B | €5.6/hour |
2x NVIDIA L40S GPU | 48GB | 724 TFLOPS | 7B LLM Inference | €2.8/hour |
1x NVIDIA L40S GPU | 48GB | 362 TFLOPS | Image & Video Encoding (8K) | €1.4/hour |
8x NVIDIA L4 Tensor Core GPU | 24GB | 1,936 TFLOPS | 70B LLM Inference | €6.00/hour |
4x NVIDIA L4 Tensor Core GPU | 24GB | 968 TFLOPS | 7B LLM Inference | €3.00/hour |
2x NVIDIA L4 Tensor Core GPU | 24GB | 484 TFLOPS | Video Encoding (8K) | €1.50/hour |
1x NVIDIA L4 Tensor Core GPU | 24GB | 242 TFLOPS | Image Encoding (8K) | €0.75/hour |
NVIDIA P100 | 16GB | 19 TFLOPS | Image / Video Encoding (4K) | €1.24/hour |
Option | Value | Price |
---|---|---|
Zone | Paris 2 | |
Instance | 1x | 0€ |
Volume | 10GB | 0€ |
Flexible IPv4 | Yes | 0.004€ |
Total | |
---|---|
Hourly | 0€ |
Daily | 0€ |
Weekly | 0€ |
Monthly | 0€ |
Kubernetes Kapsule to optimize costs
Deploy and Scale your infrastructure with Kubernetes.
Object Storage to store and prepare your data
Deploy models with NVIDIA Triton Inference Server on Scaleway Object Storage
Docker AI Image to speed up your task
Launch the container of your choice step by step.